The SuperImageDenoiser, or short, SID! For a long time it was annoying and difficult to use, but now it is a super easy to use add-on that allows you to denoise your images super cleanly with minimal loss of detail! If you want to use external programs such as AfterEffects, it can also export Multilayer-EXR files, which others can't! Anyone who takes photos will need Super Denoising for Mac. Super Denoising for Mac.
Download PDFAbstract: Super-resolution and denoising are ill-posed yet fundamental imagerestoration tasks. In blind settings, the degradation kernel or the noise levelare unknown. This makes restoration even more challenging, notably forlearning-based methods, as they tend to overfit to the degradation seen duringtraining. We present an analysis, in the frequency domain, ofdegradation-kernel overfitting in super-resolution and introduce a conditionallearning perspective that extends to both super-resolution and denoising.Building on our formulation, we propose a stochastic frequency masking ofimages used in training to regularize the networks and address the overfittingproblem. Our technique improves state-of-the-art methods on blindsuper-resolution with different synthetic kernels, real super-resolution, blindGaussian denoising, and real-image denoising.
Submission history
From: Ruofan Zhou [view email][v1] Mon, 16 Mar 2020 11:21:20 UTC (7,320 KB)
Super Denoising Software
[v2]
[v3]Thu, 23 Jul 2020 15:26:52 UTC (5,802 KB)
Full-text links:
Download:

Super Denoising
References & Citations
Super Denoising Software
arXivLabs is a framework that allows collaborators to develop and share new arXiv features directly on our website.
Both individuals and organizations that work with arXivLabs have embraced and accepted our values of openness, community, excellence, and user data privacy. arXiv is committed to these values and only works with partners that adhere to them.
Have an idea for a project that will add value for arXiv's community? Learn more about arXivLabs and how to get involved.
Super Denoising
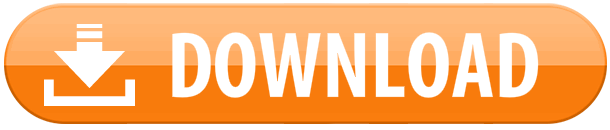